What is big data and how does it work?
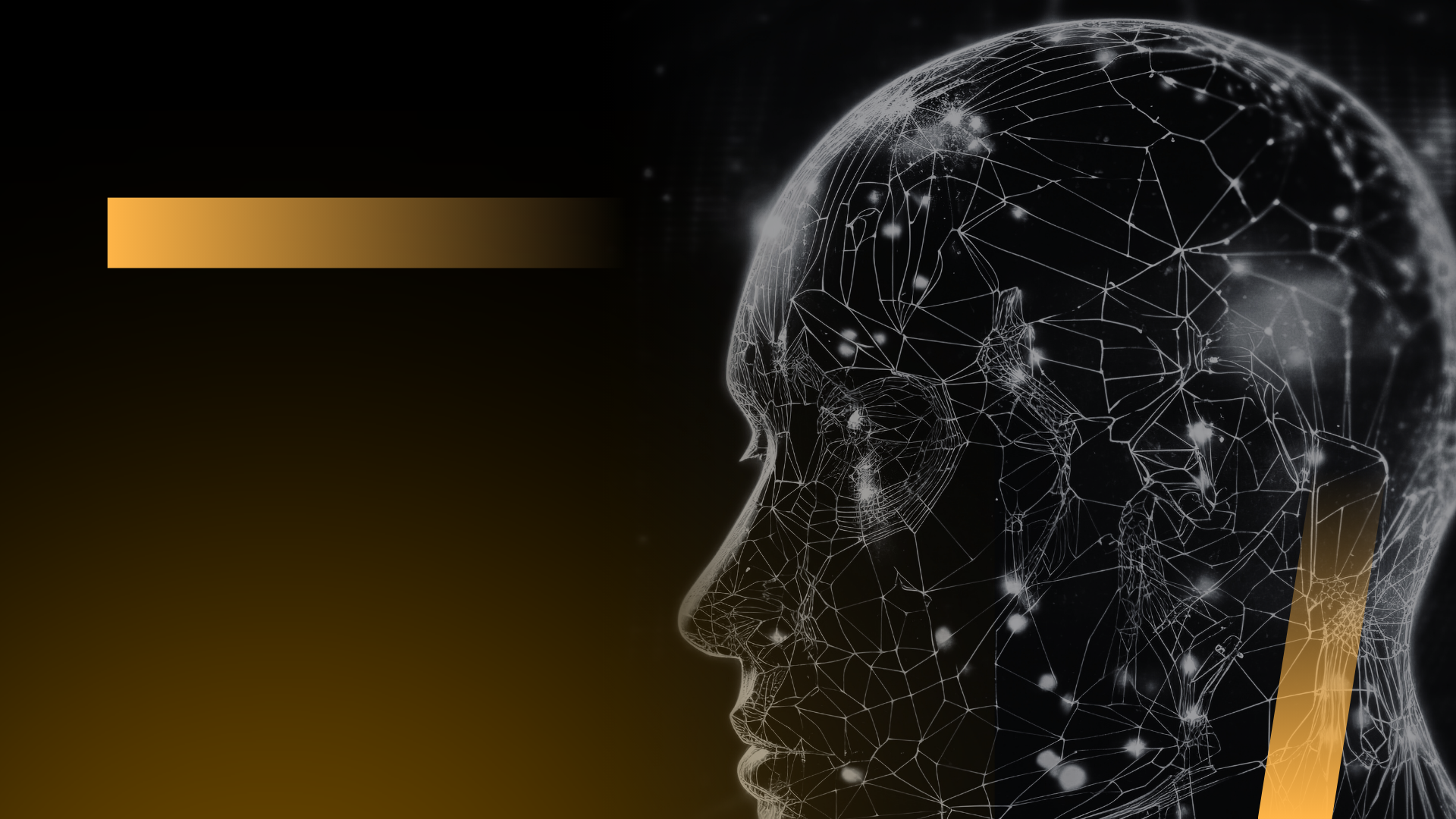
‘Big data’ is a phrase that we’ve become used to hearing in everyday life, and its sizeable handle is certainly justified.
To put the scale of modern data into perspective, consider this: every day, platforms like Facebook manage over 4,000 terabytes of new information. It’s enough to justify entire data centres dedicated to just one company — and it brings massive insights if used properly.
But what is big data? And how does it really work? For businesses, the ‘big’ in big data doesn’t just detail its overwhelming size, it also describes its transformative potential. When companies use these mountains of information, they can uncover insights that drive better decisions and justify strategic moves.
In this blog, we'll explore big data's intriguing history, its undeniable significance in modern life and the pivotal role it can play in boosting decision-making in your business.
Big data: An introduction
The term ‘big data’ is a concept rather than an accurate description. It refers to an amount of information that is beyond the capability of traditional databases and software tools to capture, manage and understand. But big data doesn’t always refer to the sheer size of storage space used, it's also about the complexity and speed involved in data generation.
The meaning of big data is also relative to the hardware capabilities and knowledge of a time period. For that reason, it’s helpful to learn about its early days first.
The history of big data analytics
While the concept of big data might seem recent, our journey with managing large data sets began decades ago and has continually evolved.
Data collection and analysis have roots in the 1960s and '70s with the advent of data centres and relational databases. As the internet gained widespread use, and data generation exploded, new methods were invented to store and use this information. This was the era when platforms like Hadoop emerged as critical solutions for handling large amounts of data.
Nowadays, our constantly connected lives create enormous amounts of data. Internet-of-Things (IoT) devices, especially, mean we’re always creating new data points, even when we don’t realise it. Estimates show that we make around 120 zettabytes each day — roughly 60 times more than in 2010.
This sheer amount of data processing requires highly efficient and reliable data management — that’s where the three Vs come in.
Understanding the three Vs: Volume, variability, and variety of data
The three Vs of big data are an excellent way to appreciate its depth and complexity.
- Volume: This represents the immense quantity of data accumulated from diverse sources like smart devices, social media, and industrial equipment.
- Velocity: Refers to the rapid rate at which data is generated. This is especially applicable to today’s real-time data-driven sources like sensors and IoT devices.
- Variety: Highlights the diverse formats of data. From structured numerical entries in databases to unstructured formats like videos and emails, all raw data points have their own needs and best practices.
For the past two decades, the three Vs have highlighted the challenges and uses of big data. But as we gain a greater understanding and technology improves, we see even further advancements.
What is the state of big data now?
As we mentioned, the term ‘big data’ is relative. In its early days, big data might have meant information exceeding a gigabyte. Now, we’re handling and analysing petabytes and even exabytes of data —thousands or millions of times larger than before.
Faced with so much information, it’s essential to emphasise that quality matters just as much, if not more, than quantity. The scale of big data has expanded exponentially. But its usefulness in data mining and predictive analytics hinges on the quality of information held within our databases and file storage.
For example, deep learning – a subset of machine learning transforming almost every industry – works best with vast datasets. The more high-quality data you feed it, the more accurate and insightful the results. Essentially, it's about using extensive data to run algorithms and train artificial intelligence (AI) models efficiently.
For this reason, two more Vs have been added to our repertoire: veracity and value. The true value of data is realised when it's both insightful and reliable, letting us make informed business and life decisions.
Use cases: Who should use big data?
So, which businesses should use big data solutions? While there are many potential applications, big data is especially helpful for companies looking to:
- Streamline product development to learn what customers want
- Improve the customer experience from purchase to support
- Avoid downtime, using sensor data streams to predict equipment failures before they happen
- Use automation to improve repetitive or error-prone tasks
- Generally increase operational efficiency by learning more about their business
Advancements in technology have revolutionised the way we look at data storage. Cloud and data centre storage is now both more affordable and efficient. This means businesses can store more data cost-effectively, building massive data lakes and warehouses for better analysis than ever.
How big data works
Gone are the days of predictable, traditional data formats. Businesses now handle many types of data, from structured spreadsheets to tweets and images. These broad and varied data sources mean that information must be stored properly to allow for advanced analytics.
Structured and unstructured data
A fundamental difference between data types is whether it’s structured or unstructured.
Structured data is generally:
- Uniform, predictable, and orderly
- Numerical and factual
- Much easier to probe and decipher for definitive insights
Unstructured data, on the other hand, can be much more challenging to manage. It’s generally the opposite of structured data, being of diverse sources and not strictly formatted. It can cover non-numerical elements like pictures, blocks of text, and multimedia. Analysing unstructured data usually requires advanced tools like natural language processing (NLP) and unsupervised AI algorithms.
It’s possible to combine both into one data type, known as semi-structured data. A good example is an image. The image data is unstructured, while the metadata of name, file type and mobile device is structured.
While structured data is clear-cut and logical, unstructured data is more of a mixed bag that requires intricate handling. Once you’ve understood your data type, you can begin the rewarding process of big data analysis.
The benefits of using big data analytics
Modern businesses know that data is power. This shift is mainly due to the remarkable advantages of big data analytics, learning key insights from the vast information our systems gather. If stored, processed, and analysed correctly, big data can bring companies many benefits, such as:
- Decision-making backed by real-world insights: Companies aren't just looking at themselves for answers. Now, external data, particularly from social media sites like Facebook and Twitter, plays a pivotal role in helping businesses manage their approaches.
- Business intelligence: The characteristics of big data can tell companies a lot about future performance. Whether forecasting or improving services, data science can improve a company’s operations.
- Meeting customer needs: We no longer rely on paper feedback forms. With the amalgamation of big data and AI algorithms, understanding customer sentiment and improving their experiences has become a more intuitive process. Companies can also find correlations between customers’ needs to perfect their products.
- Cost savings: With the proper hardware setup, companies can profit from their data many times over. Many choose to work with a managed cloud services provider to benefit from their cost-effective storage and computing resources.
Are there any downsides?
Of course, companies should take care when implementing big data into their business processes. While big data analytics offers fantastic opportunities, it isn't without its challenges:
- Navigating through structured and unstructured data demands a particular set of skills. Finding data engineers or data analysts within budget can sometimes be a challenge.
- With vast amounts of data comes the imperative need for robust privacy and security measures. Depending on the industry, there may also be regulatory needs.
- Not all data is good data. Sifting through to ensure quality can be a daunting task — the maxim Garbage In, Garbage Out is especially applicable to big data.
- With different data types, you need the right storage. Ensuring the best hardware infrastructure is in place is crucial.
In many cases, it can be helpful to speak to a cloud services expert to help you address these challenges. This will maximise the ROI from your big data insights.
How Nasstar can help
While big data has come far, its usefulness is only getting started. Cloud computing has expanded possibilities even further through almost infinite scalability, with platforms that allow data scientists to spin up ad hoc datasets and test new theories.
We’re also learning and designing increasingly intelligent models that help us understand unstructured data. This is key to making better business decisions.
At Nasstar, our professional IT services can help businesses of all sizes embrace the power of big data. Our goal is always to drive business value, with our teams working backwards from your unique challenge or opportunity to find a viable and effective solution. Speak to a specialist to learn more about your possibilities.
Frequently Asked Questions (FAQs)
What is big data technology?
Big data technology is a collection of hardware, tools, frameworks, and techniques that help companies process and analyse large volumes of information. In a real sense, big data just describes data that's too vast for traditional databases. The goal of the analysis is to extract valuable insights from these complex and varied datasets.
Where is big data stored?
Big data is primarily stored in two places: data lakes and data warehouses.
- Data lakes hold raw, unprocessed, and unstructured information, which is more complicated — but often very rewarding — to analyse.
- Data warehouses contain processed and structured data that’s typically more ready for analysis.
How is big data used in business?
Businesses use big data to improve decision-making and predict trends. It can help in personalising customer experiences and improving operational efficiencies while also giving clues for new products or pricing strategies, for example. By analysing vast amounts of data, companies can identify new opportunities and reduce risks.