Ethical Considerations in AI: Exploring the Boundaries of Artificial Intelligence
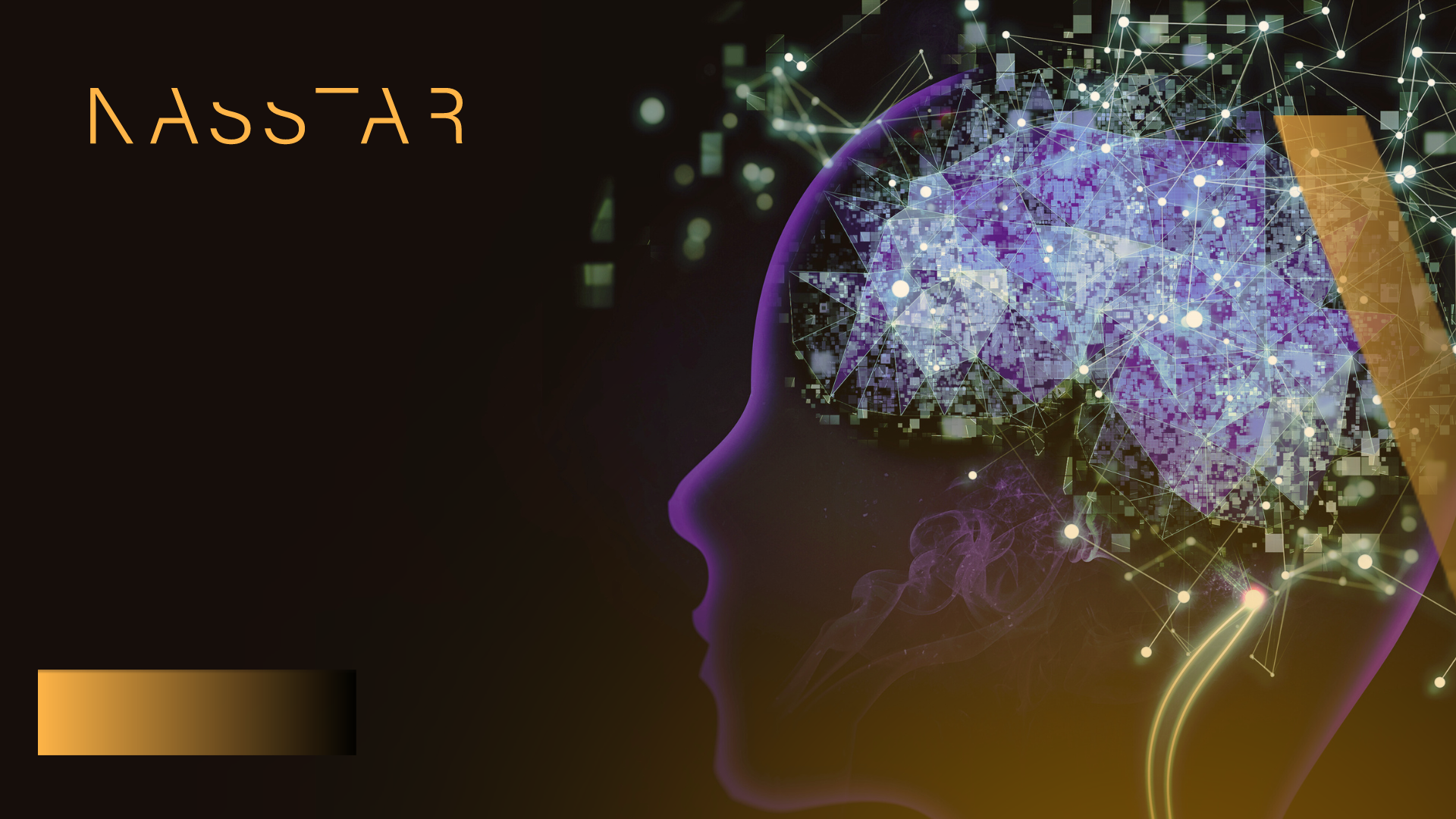
This blog has been expertly reviewed by Jason Vigus, Head of Portfolio Strategy at Nasstar and Andrea Rosales, Data Scientist at Colibri Digital.
In the ever-expanding universe of artificial intelligence (AI), many of us are eager to start using this technology and experiencing its benefits in our daily work. However, in this haste, some businesses are falling foul to unforeseen circumstances in their application of AI.
Of course, most organisations are using generative AI to improve business processes, and the intentions are positive. The problems occur when there is a lack of consideration for AI morality and ethics ahead of implementation.
AI ethics refers to a set of values, principles, and techniques that outline widely accepted standards of right and wrong. Without this, there is little guidance on moral conduct in the development and use of AI technologies. And this could cause all kinds of problems.
In this blog, we’ll discuss AI ethics in depth, considering why it’s important and how businesses can ensure they are using ethical AI practices when deploying AI technologies.
Why is ethical AI important?
The ethical implications of the rapid deployment and development of AI cannot be overstated. As this technology becomes increasingly integrated into various aspects of our lives, from healthcare to criminal justice systems, and of course businesses, understanding and addressing artificial intelligence ethics is paramount.
Jason Vigus, Head of Portfolio at Nasstar, commented: “In the blueprint of effective AI, ethics should never be an afterthought – it’s the cornerstone we lay before development begins.”
Bias and fairness
Unsurprisingly, one of the most pressing concerns surrounding AI is the perpetuation of bias within its algorithms. We know that AI systems learn from vast amounts of data. But if this data is biased or incomplete, it can lead to discriminatory outcomes.
For example, biased algorithms in hiring processes may disproportionately favour specific demographics and exclude others, perpetuating systemic inequalities.
Ensuring fairness in AI systems means businesses must pay meticulous attention to the data used for training the AI. There must also be a process for ongoing monitoring, enabling the detection and mitigation of any biases quickly to ensure damage limitation.
Privacy concerns
There have been significant privacy concerns raised due to AI’s reliance on large datasets. From facial recognition technology to personalised advertising algorithms, AI systems regularly collect and analyse personal data.
Without proper and robust safeguards, this data collection and analysis could infringe upon individuals’ privacy rights and even lead to exploitation in severe cases. Ethical AI frameworks need to prioritise the protection of user privacy through transparent data practices, strong security measures, and user consent mechanisms.
Accountability and transparency
With AI systems making increasingly impactful decisions, we must understand how those decisions are reached and who bears responsibility for them. Transparent AI algorithms allow for greater scrutiny and accountability, enabling stakeholders to understand the reasoning behind AI-generated outcomes.
When developing and deploying AI technology, it’s also essential that clear lines of responsibility are established to address any harm caused by AI systems, whether unintentional or otherwise.
How can biases in AI algorithms be addressed?
Biases in AI algorithms are formed due to two things: incomplete data or complete data with human bias. AI biases can therefore only occur due to the prejudices of humans.
Andrea Rosales, Data Scientist at Colibri Digital, commented: “It’s important to note that these biases can be introduced at various stages of the AI development process. This includes data collection, preprocessing, feature selection, and algorithm design.”
However, addressing biases in AI algorithms isn’t as simple as cleaning up your data and hoping it’s unbiased when the AI uses it for learning. Likewise, it’s naive to think removing protected classes such as sex or race could work to remove bias.
“AI's true potential lies in its ability to amplify human progress, not replicate our biases. By prioritising ethical considerations and actively addressing bias in algorithms, we can guide the future of AI towards a more equitable and just future.” said Jason.
A multifaceted approach involving both technological and sociocultural interventions is required to effectively mitigate biases in AI algorithms and ensure responsible innovation. Here are some key tips for addressing these biases:
Gather diverse and representative data
It’s essential to ensure training data is diverse and representative of the population it seeks to serve. This involves actively seeking data from underrepresented groups and continuously evaluating and updating datasets to prevent the reinforcement of existing biases. ML algorithms can change as they learn or as training data changes, so it’s vital that ongoing monitoring is conducted to prevent bias.
Ensure AI algorithms are transparent
Ensuring your AI algorithms are transparent and interpretable can help to identify and address biases. To enable this transparency, businesses should look to prioritise ‘explainable AI’. Explainable Artificial Intelligence (XAI) allows humans to understand how algorithms make decisions so they can trust the results created by the AI and better identify biases.
For greater transparency from the beginning, businesses should document their models and ensure their design and limitations are detailed. It’s also a good idea to use open-source development for even wider scrutiny.
Test early on
Implementing robust testing procedures to detect biases during the initial development and deployment phases of AI systems can help to address bias. Businesses can use specialist techniques to help them identify and mitigate biases in algorithms to ensure more equitable outcomes.
One such technique is ‘fairness-aware machine learning’ which is a subfield of machine learning designed to create models that make unbiased decisions. It seeks to reduce or completely remove discriminatory biases in predictions, especially those related to race, gender, age, or socio-economic status.
Promote diversity in AI dev teams
When developing AI solutions, encouraging diversity within the AI development teams can help to uncover blind spots and biases that could otherwise go unnoticed. Businesses should also engage with diverse stakeholders throughout the process to gain valuable insights into other potential biases and their implications.
Improve human-driven processes
When building models and evaluating their effectiveness, businesses will often uncover biases that have gone unnoticed for a while. Instead of ignoring these biases and inputting them into the training data, either consciously or inadvertently, businesses can instead identify these biases and use them to understand the reasons for them.
Using this knowledge, organisations can implement training, improve process design, and undertake cultural changes that improve their human-driven processes by eliminating bias. With human biases reduced, AI algorithm bias is much less of a concern.
Use a critical secondary AI
Alongside a human-based approach to checking AI work for accuracy, it’s a good idea to also use a secondary AI tool. This tool would have an exclusive role of analysing the outputs from the primary AI system, with the purpose of identifying and correcting, or flagging any detected biases. It’s important to police AI in this way, particularly when checking results.
Andrea added: “It’s also important to regularly audit and monitor models to assess the performance of AI models in terms of their fairness and bias. AI models should also be created to be interpretable and provide explanations for their decisions, enabling stakeholders to understand and interpret the reasoning behind each prediction.”
AI Ethics at Nasstar and Colibri Digital
Addressing the ethical considerations in AI is not only a moral imperative but also essential for building trust and ensuring responsible development and deployment of AI technologies. By tackling issues such as bias, privacy concerns, and accountability head-on, we can start creating AI systems that benefit both business and wider society.
Here at Nasstar and Colibri Digital, our teams have spent time researching and understanding AI technologies, making the mistakes, and learning from them so you don’t have to. We can confidently support you with your AI ambitions, ensuring any project you undertake factors in the relevant ethical considerations in AI.
Speak to our team about emerging technologies and ethical AI practices today.
FAQs
What are the key challenges in implementing ethical AI practices?
The key challenges in implementing ethical AI practices include:
- Ensuring the quality and representativeness of training data
- Ensuring training data is unbiased
- Ensuring algorithms are transparent and can be interpreted effectively
- Ensuring privacy is preserved, particularly in relation to individual privacy rights
- Ensuring ethical decision making by AI is maintained to ensure compliance
- Ensuring regulatory compliance and establishing regulatory or legal frameworks
- Ensuring bias in AI workforce and culture is eliminated from the beginning
How can businesses ensure transparency in their AI systems?
To ensure transparency in AI systems, business should ensure they are documenting the decision-making processes of AI algorithms, alongside explanations for their outcomes. They should also develop ethical frameworks for AI use that align with their values and regulatory requirements. Regular audits and evaluations should also be undertaken to help identify bias, errors, or potential risks.
What ethical frameworks guide AI development?
At the moment, there is no ‘one-size-fits-all' ethical framework for AI development. Instead, various ethical frameworks are being built and adopted by different communities, with a range of organisations participating in the development of an ethical framework for AI.
The academic community has also leveraged the Belmont Report to guide ethics in experimental research and algorithmic development. The three main principles of respect for persons, beneficence, and justice are being used to guide ethical algorithmic design.
How do AI ethics intersect with privacy concerns?
AI and the gathering of data has posed data privacy risks. Navigating this crossover between the two should revolve around respect for individual autonomy, consent, and transparency. AI systems need to operate in a way that respects privacy and the dignity of individuals, including how data is collected and whether people have consented to this.
Are there regulations or guidelines governing AI ethics?
There are currently several regulations and guidelines governing the use of AI and its ethical considerations. These include the following:
- The UK Government – AI Code of Conduct
- The World Health Organization – Ethics and governance of artificial intelligence for health: Guidance on large multi-modal models
- The European Commission – Ethical Guidelines for Trustworthy AI
- The European Union – GDPR
- The World Economic Forum – The Presidio Recommendations on Responsible Generative AI
- The Association for Computing Machinery – Ethical Guidelines and Principles in the Context of Artificial Intelligence
- The Organisation for Economic Co-operation and Development – OECD AI Principles
There are also several other country or region-specific guidelines that can be used as guidance when considering ethical AI practices.